Last updated 23 Jun 2023
Tuning, in the context of voice biometrics, refers to the process of adjusting the configuration and parameters of a voice biometric system to optimize its performance for a particular task or environment.
Voice biometric systems use machine learning algorithms trained on generic datasets to recognise and differentiate individual voices. Tuning in this context refers to optimising system performance by determining the optimal algorithm for comparison, identifying the optimal configuration of any audio pre-processing steps and augmenting the existing training with data from the actual operating environment to improve discrimination between speakers and optimise overall performance. Tuning generally involves several steps:
- Data Collection – Collect a representative sample of verification and enrollment utterances from the operating environment. This should contain multiple verification utterances for each speaker and ideally take place over a significant period to allow for natural variation in the speaker’s voices and channel usage.
- Training and Testing – Part of the data set is used to augment or, in some cases, replace the Voice Biometric model’s existing training, often producing a custom Background Model (BGM). This new model is evaluated using different sample data to understand its performance with a True User Impost Test (TUIT). Other parameters, such as different minimum enrollment and verification audio lengths and audio processing configurations, such as signal-to-noise ratio, are also evaluated to understand the impact on performance. This may also include evaluating different detective measures, such as synthetic speech detection.
- Decision – Test data is reviewed, and a decision is made on the optimal biometric threshold and other key configuration parameters based on the implementing organisation’s risk and performance objectives.
- Implementation – The new model and updated configuration are implemented into production, which may require retraining existing speakers against the new model using their original enrolment audio.
Related Terms
Popular Posts
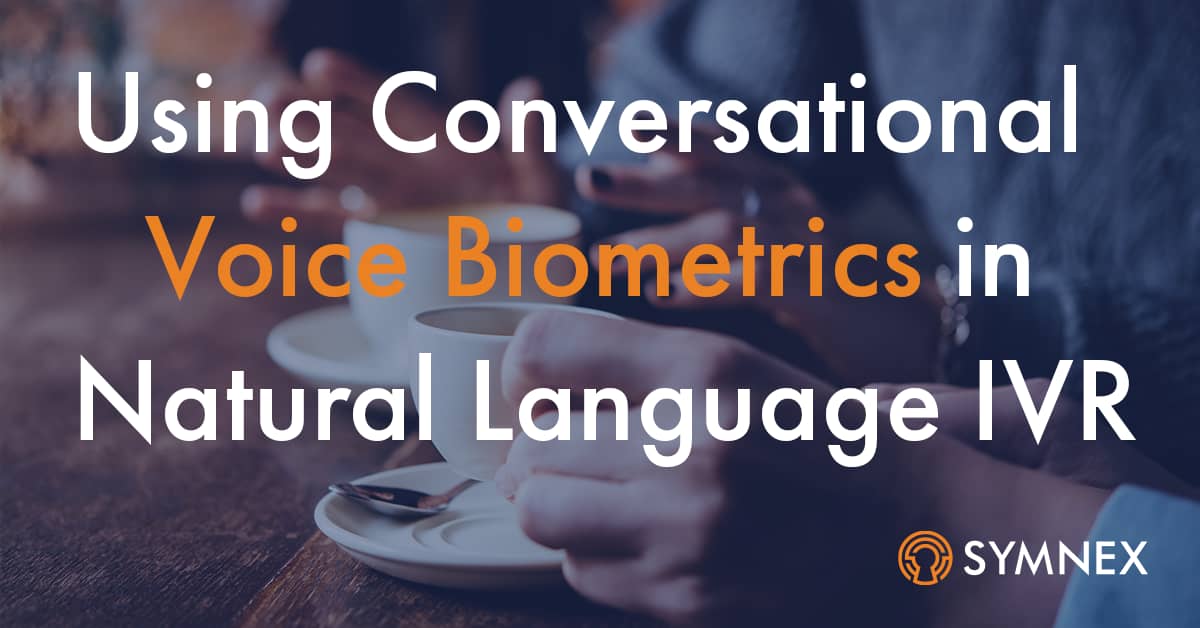
9 mins read
Advances in Speaker Recognition technology and increasing acceptance of conversational interfaces are allowing organisations to authenticate callers using the short utterances now obtained in natural language and speech driven self service applications, without the overhead of traditional active enrolment processes.

4 mins read
Our Call Centre Security Experience Scorecard gives you the benefit of our 10 years of experience in just 10 minutes. It helps organisations understand how to improve the usability, efficiency and security of their experience.
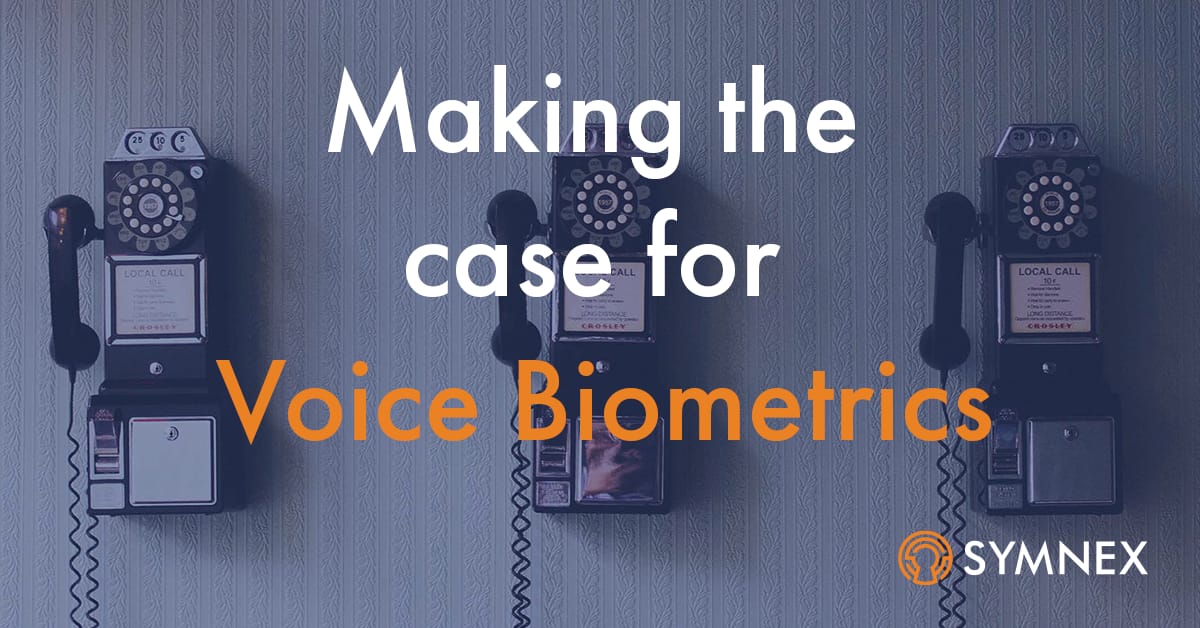
4 mins read
Nearly all implementations of voice biometrics are driven by promises to make it easier for customers to gain account access, reduce the time spent by front-line agents in authenticating customers, and making sure customers’ personal data and services are secure.